The Pandemic Recession, part 1: Pre-Pandemic
We will look at the state of the ‘economics of pandemics’ prior to the Covid outbreak. This will include some theory about when to intervene to reduce infections while minimising disruption, some empirical work on how well interventions actually reduce the spread of infections, and some predictions about the kinds of recessions that pandemics are likely to cause.
The Economics of Viruses: Pre-pandemic Theory
Epidemiology –the study of the distribution/spread of illness in the population– mostly uses SIR models of virus spread. Lets briefly explain how these models work so that we can then understand what economics adds to these models. In a SIR model every person in the population is in one of three states: Susceptible, Infected, or Recovered. Depending on model setup there may be a fourth state, Dead. Susceptible people catch the virus and become Infected with some probability, , this probability is increasing in the number of infected. Infected people are ’resolved’ with some probability,
, and when resolved they immediately become either Recovered with some probability,
or otherwise die (with probability
). Note that while the SIR model is suitable for Covid-19 not all viruses fit this and epidemiologists also regularly use SIS, Susceptible-Infected-Susceptible (for diseases you do not get immunity to), and SEIR, Susceptible-Exposed-Infected-Recovered models.
Economic-SIR models add that people choose how much contact/socialising to do (socialising generates income or even just utility directly). The infection probability is then increasing in the amount of contact/socialising (as well as in the number of infected). So economics emphasizes the role of peoples decisions about risks/protection in the spread of virus. We can think about Economic-SIR models as adding a role for human behavior to standard SIR models.
A standard SIR model produces a ‘single wave’, as shown in Figure 1A. An infected person is assumed to infect others at a constant rate and so the number of people catching the virus increases as the number of infected increase, making the curve get progressively steeper. Eventually most people are either recovered or dead and so the number of infected people falls and the curve flattens out towards the top and then proceeds to fall. Figure 1B shows an Economic-SIR model. The behavioral responses are needed to explain ’multiple waves of infection’. High levels of infections mean people take protective action (e.g., masks and social distancing) reducing the rate of infection and causing the peaks of the ‘waves’, as infections fall people relax and cease to take protective action, leading to the trough and a new wave begins. Figure 2 shows the infections for the USA and illustrates the multiple waves in infections which emphasizes the empirical relevance of these behavioral responses. The behavioral responses have important implications for spread of the virus, and also for understanding the role of any interventions to reduce the spread of the virus.
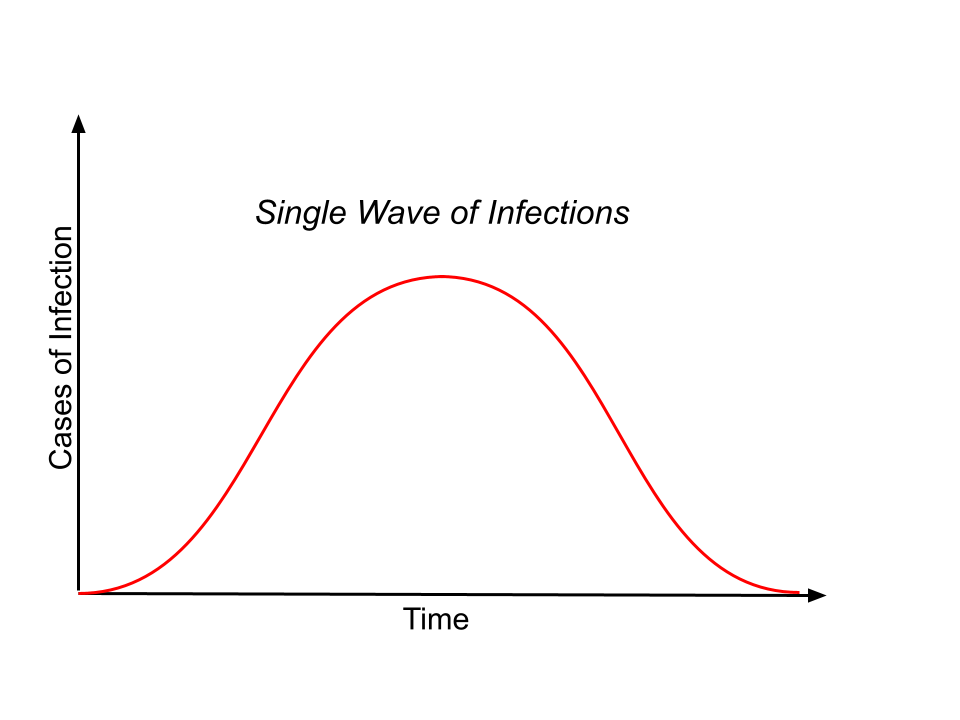
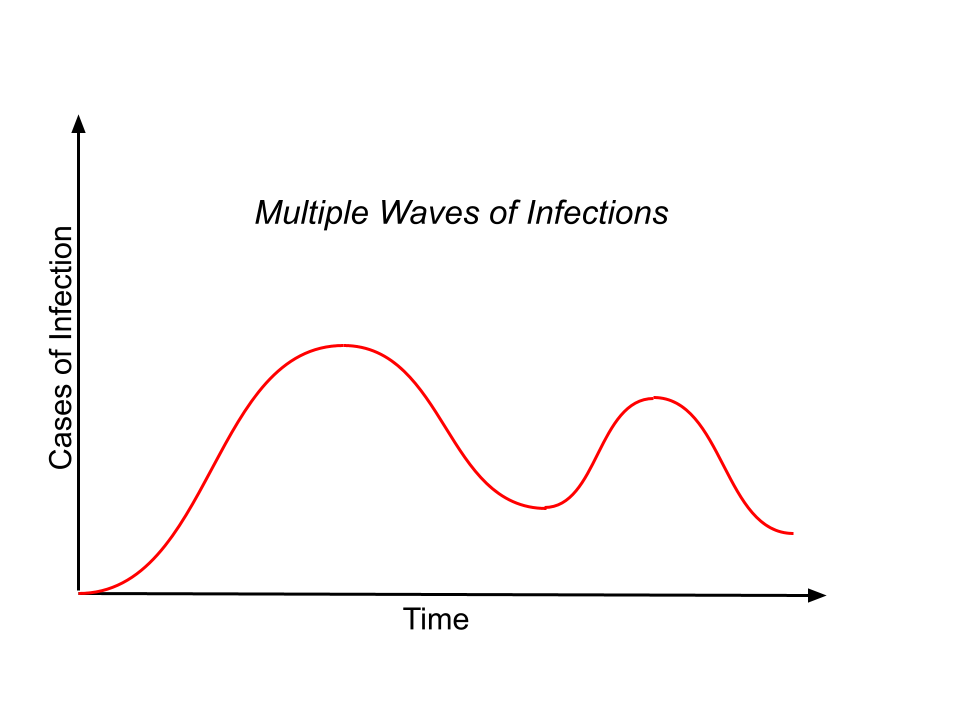
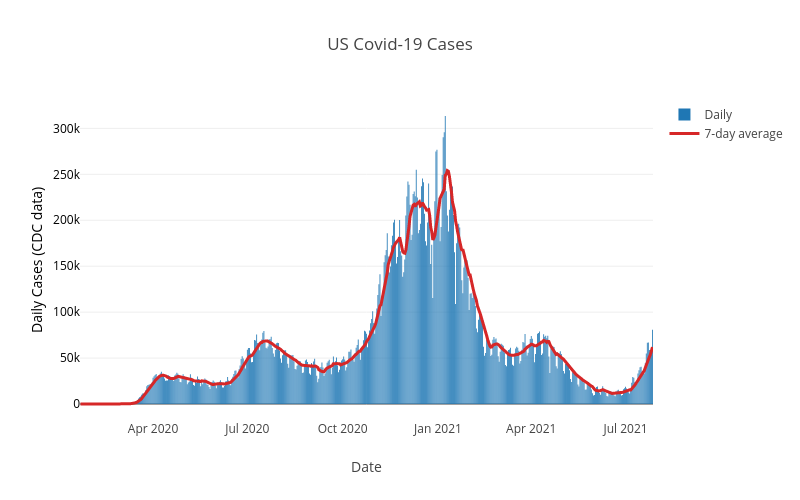
Now that we have a way to think about how viruses spread we turn to the question of when to intervene? What are the costs and benefits of early vs late intervention? Economic-SIR models have an negative externality (I use virus-protection to protect my own health, but I ignore that me not getting infected also reduces your probability of getting infected). This creates an efficiency motive for public intervention. Geoffard & Philipson (1996) and Sims, Finnoff & O’Regan (2016) look at the potential role of public interventions in stopping or slowing the spread of a virus. With certainty about the virus, early intervention is best as it is cheaper (avoiding contact, and hence infection, is costly and early intervention means smaller reductions in contact are required). With uncertainty —about, e.g., infectiousness, or death rate— there is an ’option value’: by waiting we find out information (resolve the uncertainty) which shows that the virus is infectious/deadly, in which case we do want to lockdown, or benign, in which case we do not want to lockdown. (Relies on the assumption there is a fixed-cost to intervention.) So in the real world we have competing motives for early vs late intervention. Once we understand how deadly a virus is and how quickly it spreads we are better off doing any intervention as early as possible. But when we don’t yet understand the virus we would like to wait for more information.
The Economics of Viruses: Pre-pandemic Empirics
One strand of pre-pandemic work focused on estimating impacts of interventions, e.g., school closures, on virus spread. A good example of this is Adda (2016) which uses weekly regional French data for influenza, gastroenteritis and chickenpox. It estimates the roles of school closure, transport shutdowns, and high-speed train networks in slowing/slowing/speeding the spread of virus. The opening of new high-speed train lines is found to increase the speed at which viruses spread to the newly connected regions. The case of school closures is very interesting. As expected school closures reduce the incidence of viruses among children who no longer pass them to one another in class. But surprisingly the school closures also increase the incidence of viruses…among grandparents! All the children spend their holidays visiting grandparents and taking their illnesses with them.
Another strand of pre-pandemic work aimed to answer the question: How big a recession would a pandemic cause? Two good examples are McKibbin & Sidorenko (2006) and Keogh-Brown, Wren-Lewis, Edmunds, Beutels & Smith (2010). Both model the pandemic as a shock to the economy (essentially a fall in employment and consumption) and evaluate the implication for GDP. The focus on the likely economic costs, not about evaluating different policy responses. Both suggest short/sharp recessions. A prediction that now looks prescient for the pandemic recession in 2020; we will discuss the short/sharp nature of this recession later. It is worth noting that most existing work estimating the recessions caused by pandemics was about either AIDS or SARS.
The Economics of Viruses: Past Pandemics
The Black Death in the 14th Century killed 50% of the population in England. This big fall in the labour supply resulted in higher wages and lower asset prices. But for the present pandemic even in US the death toll is well under 1% of the population. So we are not likely to see this effect in the Pandemic Recession. Plus most of the dead have been elderly and retired. The Spanish Flu of 1918 killed around 50 million in a global population of around 2 billion, so roughly 2.5% of population. In 2020 this would mean 195 million people, a number many times greater than the current death toll.
Robert,
Very nice information. You pointed out that the behavioral prediction of the SIR model depends on behavioral responses. These responses in the case of covid-19 are multidimensional and complex, some difficult to quantify their importance.
Quite different from early historical pandemics, in 2020 human society knew about the virus, and knew how to prevent its spread, and was able to prevent its spread thanks to government’s fiscal measures. But a significant portion of population appeared to have just ignored the risk of being infected, and resisted the vaccine. This can help explain why China’s lockdown was effective. In some sense, the tragedy of commons needs extraordinary actions by government.